Machine Translation (MT) is only as good as the human intelligence surrounding its implementation. When we refer to implementation, it isn’t only how we train the engines, but also how we manage the environmental impact. Teaching a machine the complexities of learning human language results in a heavy level of computation that is energy intensive. Tuning neural architecture through trial-and-error results in a larger than necessary carbon footprint. More recent estimates suggest that the carbon footprint of training a single AI is as much as 284 tonnes of carbon dioxide equivalent — five times the lifetime emissions of an average car. Therefore, efficient machine learning (ML) management is key to ultimately mitigate carbon emissions in ML. The way we train MT engines could use significantly less processing power to reduce our environmental impact which includes optimizing code, hosting cloud infrastructure in regions that use renewable energy sources, and more.
CQ fluency is proud to launch our CQtrees initiative where we commit to planting a tree for every engine we train to help offset carbon emissions. As natural carbon absorbs to clean the air, one tree can absorb up to 22lbs per year during their first 20 years of growth. Trees of course have many other benefits beyond storing carbon. They give us oxygen, stabilize soil, provide shelter/food for wildlife, regulate temperatures, slow the flow of water through landscapes, and much more. We are proud to offer this opportunity to have our employees, vendors and clients help us plant trees as a volunteer initiative and support programs that plant trees in the communities we serve (North America, South America, Europe and more).
Translation efficiency is at the core of what we do. CQ fluency has invested considerably in the research and development of AI, machine translation, process automation, and other innovative translation management solutions for our clients. With the evolution of language technology, we have strategically built nimble teams to help best integrate our evolving platforms to achieve cost, security, speed, scale, and quality goals. Our technology solutions work hand-in-hand as part of a larger ecosystem with efficient ML architectures at the heart of it. Aside from CQ fluency’s commitment to plant a tree for every engine, we train to help offset carbon emissions, our MT expertise reduces our impact to the environment. From the way we operate ML hardware to the process in which we train natural language processing (NLP) models, to the way we factor in how specific language pairs perform, we are continually building on our best practices to further reduce CQ fluency’s total energy use on behalf of our clients.
Companies like Google have also pledged to offset their carbon footprint as it pertains to machine learning through their model titled “4Ms” and is available to anyone using Google Cloud services. These four practices together can reduce energy by 100x and emissions by 1000x.
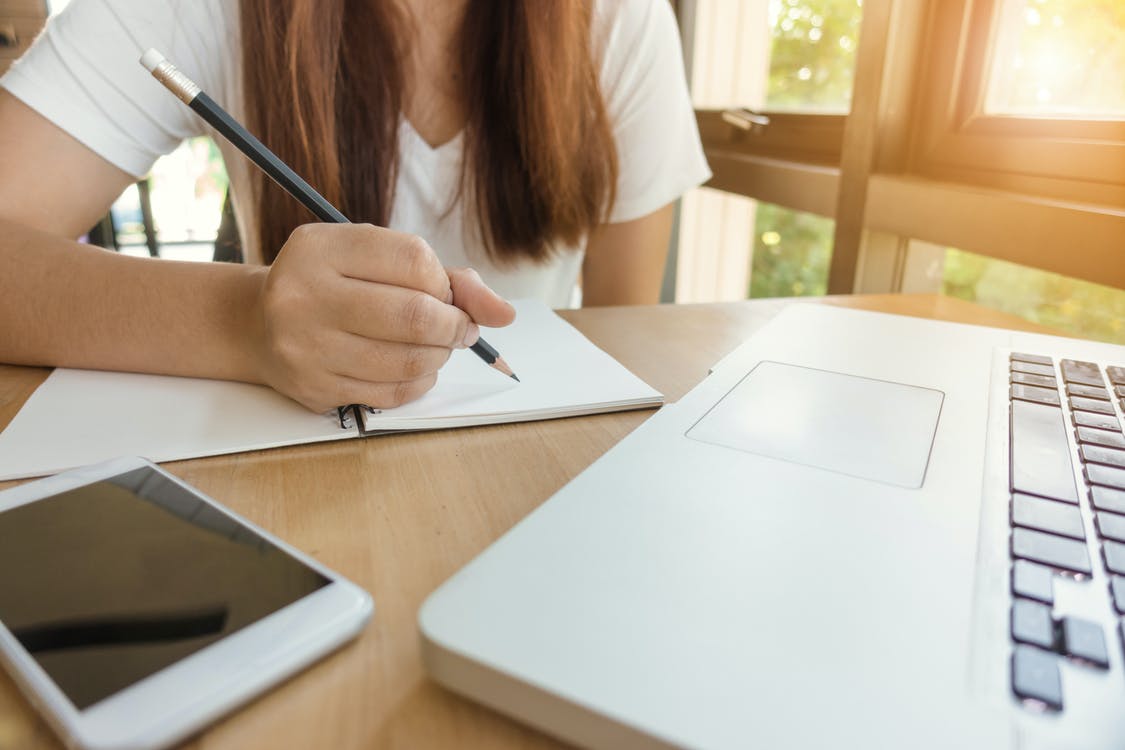
- model. Selecting efficient ML model architectures, such as sparse models, can advance ML quality while reducing computation by 3x–10x.
- machine. Using processors and systems optimized for ML training, versus general-purpose processors, can improve performance and energy efficiency by 2x–5x.
- mechanization. Computing in the Cloud rather than on premise reduces energy usage and therefore emissions by 1.4x–2x. Cloud-based data centers are new, custom-designed warehouses equipped for energy efficiency for 50,000 servers, resulting in very good power usage effectiveness (PUE). On-premise data centers are often older and smaller and thus cannot amortize the cost of new energy-efficient cooling and power distribution systems.
- map optimization. Moreover, the cloud lets customers pick the location with the cleanest energy, further reducing the gross carbon footprint by 5x–10x. While one might worry that map optimization could lead to the greenest locations quickly reaching maximum capacity, user demand for efficient data centers will result in continued advancement in green data center design and deployment.
The process of each engine training results in extensive environmental impact from the processing power needed for machine learning. That process makes sure it is essential that you are getting a trained model right the first time. At CQ fluency, we have developed pre-training assessment scorecards and proprietary scripts to optimize the data sets before training. This allows us to get first-time trained models to reach BLEU scores and edit distance rankings high enough to avoid needing a re-training with a larger dataset.
Every language service provider (LSP) can still do their part by only training engines when needed, by donating to a service, by analyzing previously trained models that required re-training to understand how they can avoid similar re-training. We all must do our part to mitigate carbon emission in ML.