Artificial intelligence (AI) translation has the potential to revolutionize healthcare by breaking down language barriers and facilitating communication between healthcare providers and multicultural patients. But AI is rampant with bias, and bias in healthcare communications can negatively impact patient outcomes. In a world that increasingly relies on AI to break down language barriers in healthcare, eliminating bias can mean the difference between effective treatment and dangerous misunderstandings.
AI is gaining in usage because it promises lightning-quick translations, but unless we address its biases what we gain in translation efficiency we might lose in cultural sensitivity and accuracy, and the risks are significant.
Healthcare organizations are on board with this: 28 of them recently signed a voluntary commitment to support the safe development of AI.
Farhanna Sayegh, Executive Director of Multicultural Marketing at CQ fluency says, “When bias shows up in translations in health care or medical settings, patients and customers can be harmed. For example, if instructions or treatment plans are mistranslated or are not culturally sensitive then that can be really dangerous for a patient. The language services industry needs to tackle this problem to make sure that automated translations do not contain bias in any form.”
Let’s explore several unintended consequences of bias in AI-generated content and translations and look at how we can begin to move towards cultural sensitivity in AI communications.
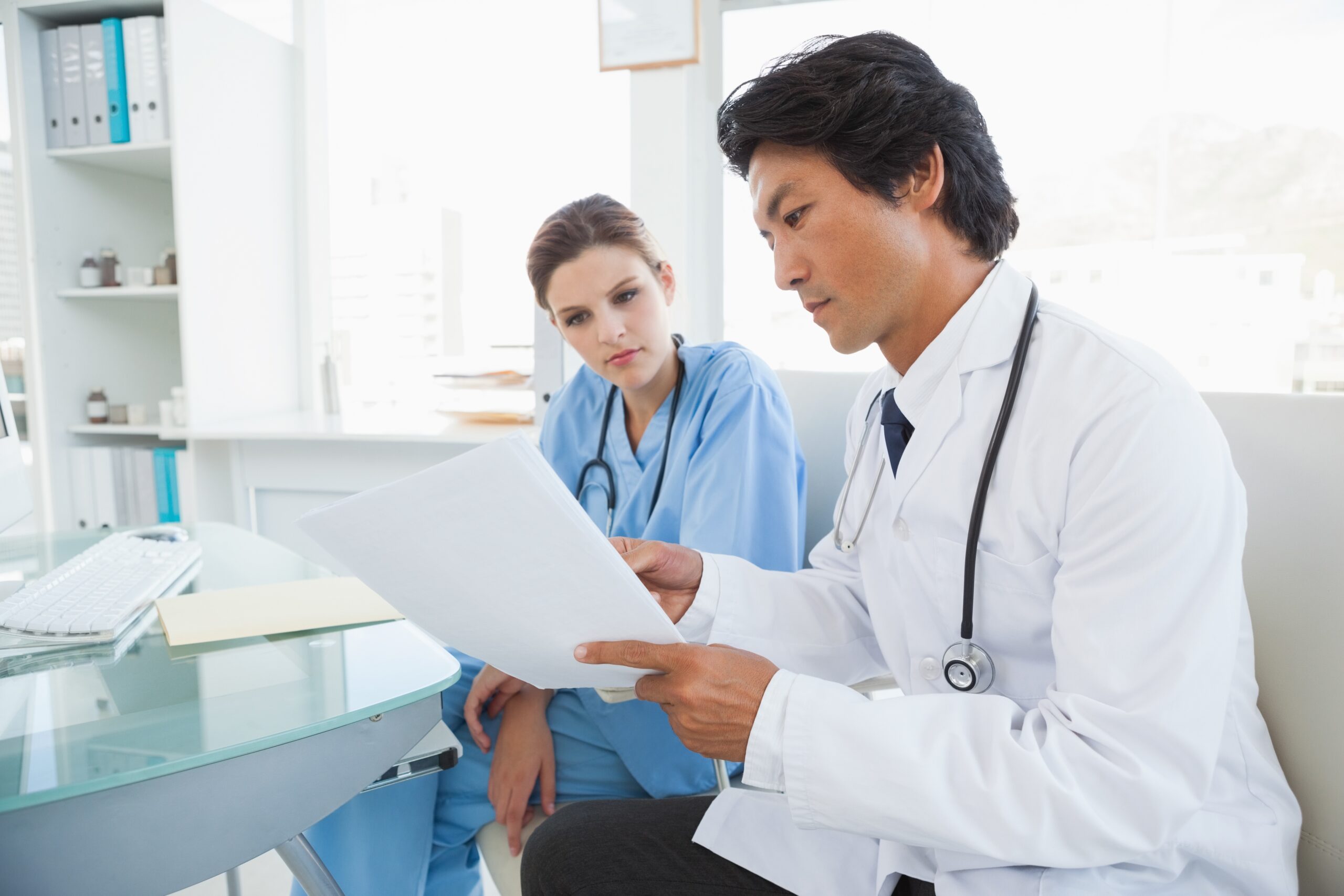
inequitable patient outcomes
In the United States, people who speak English as a second language have poorer health outcomes than native English speakers do. Lack of health literacy is a significant part of the problem, and cultural bias in translated information is a contributing factor.
For example, during the COVID-19 pandemic, translated fact sheets about the illness often recommended that people call 911 if they noticed “bluish lips or face” in affected individuals. But this advice is geared toward people with lighter skin tones, so the COVID-19 Health Literacy project changed the wording from “bluish” to “discolored.”
Cultural competence in communication is also an important part of encouraging health plan members and patients to maintain their health with preventative care. For example, by using cultural insights to promote colon cancer home screening tests, a global healthcare organization achieved a return rate 32% higher than the U.S. Colon Cancer Screening Program. This would not have been possible with AI alone.
compromised clinical trial integrity
Clinical trials historically suffer from a lack of diversity, which means that results may not apply to different racial and cultural groups. Also, biased AI translations can result in non-diverse or skewed clinical trial recruitment, leading to trials that don’t accurately represent the target population. Language barriers and lack of trust are key obstacles.
Research shows that diversity in recruitment can be improved by “personalizing outreach and recruitment to specific groups’ beliefs and values and aligning recruitment messaging with language preferences and motivations for study participation.” However, if your recruitment materials are biased against specific groups of people, those people are less likely to trust your organization with their health.
This compromises the validity and applicability of the trial outcomes and can lead to problems for patients down the line. For example, some anti-epilepsy drugs can lead to severe skin reactions that are more common in Asian patients due to genetic factors. If most of the participants in a trial are Caucasian, reactions and side effects like this go unnoticed until the drug is on the market.
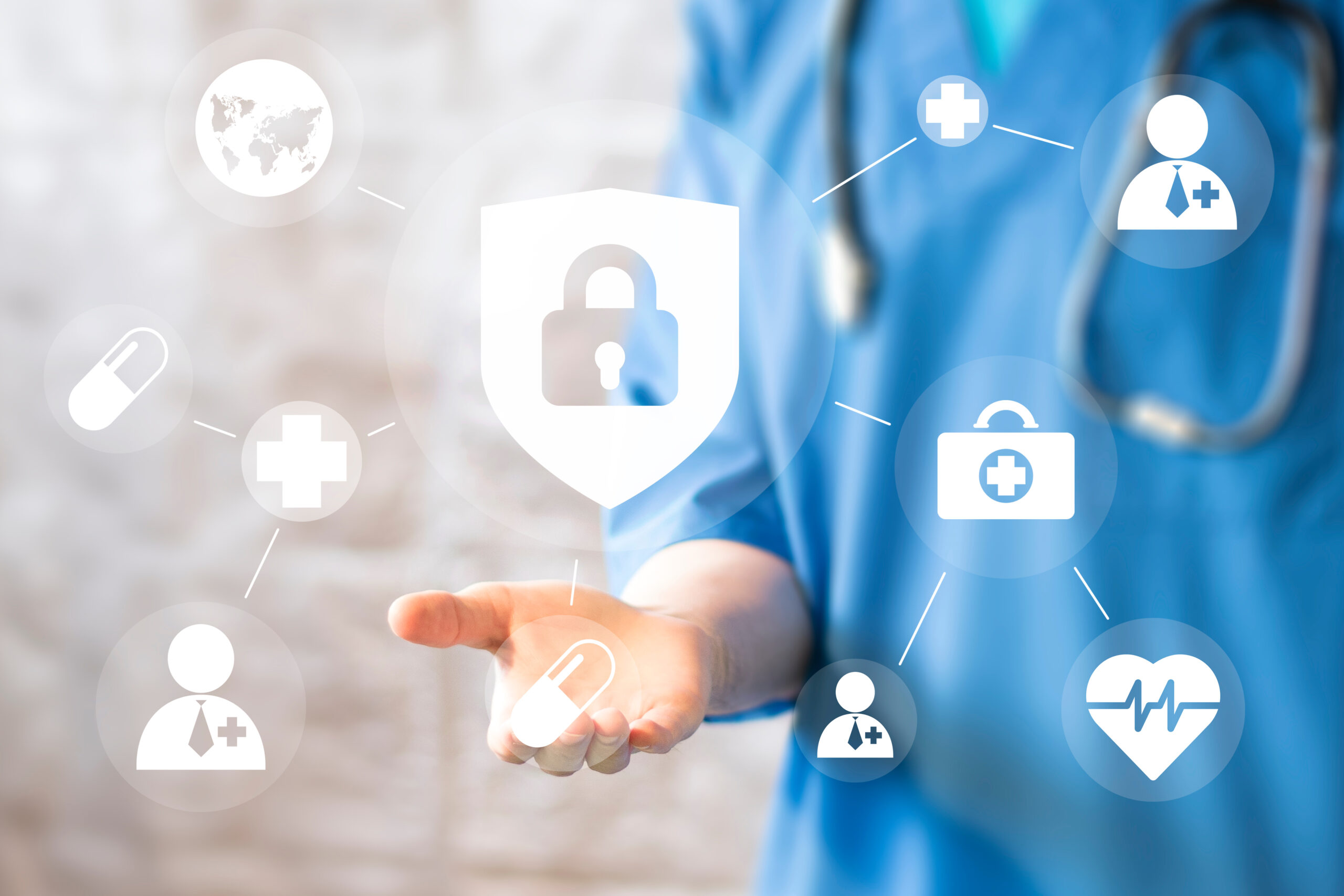
compliance issues
In the highly regulated health sector, biased content can result in non-compliance with regulations, potentially leading to legal challenges and fines.
For example, new regulations in the US require diversity plans in clinical trials, but recruiting a diverse patient population for these trials remains challenging. Cultural relevance in communication is key to building trust and participation in multicultural, multilingual patients. Presenting them with biased or insensitive translations will discourage them from taking part.
Other regulations, like Title VI of the Civil Rights Act, require recipients of federal financial assistance to take reasonable steps to ensure their programs, services, and activities are accessible to eligible people who speak English as a second language. If AI produces a translation that’s biased to the point of causing misunderstandings, that could lead to a violation.
perpetuated inequities and prejudices
AI doesn’t just reflect our biases, it perpetuates them. New research suggests that humans can unconsciously absorb the biases they see in AI-generated content and that this bias can persist even after they stop using AI tools.
That’s why it’s essential that we act to clean up biased AI data. As people and organizations shift toward using AI for communications-related tasks, we must take steps now to address the problem of bias in AI before it becomes a self-perpetuating cycle.
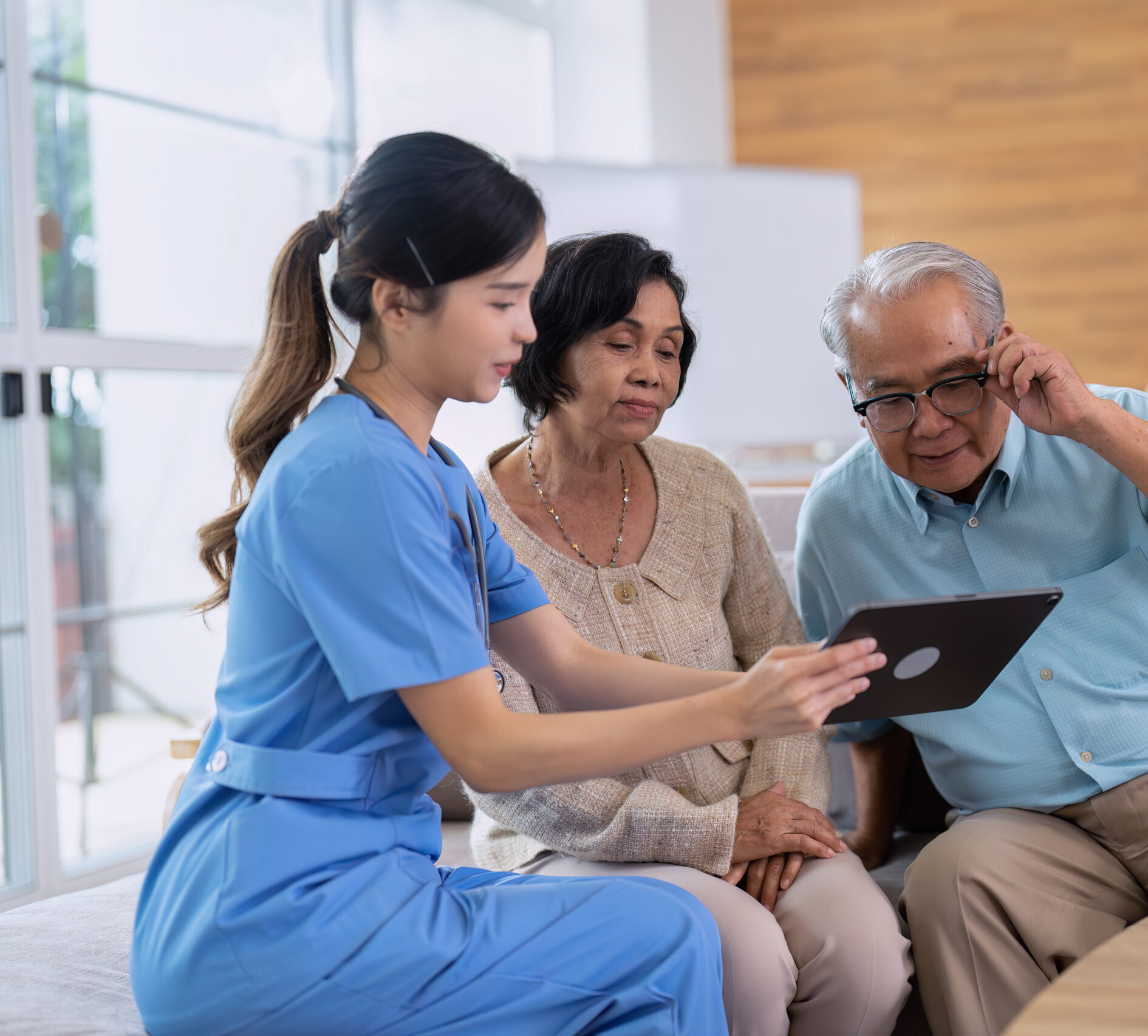
lack of patient or consumer trust
Bias in healthcare information can undermine trust in healthcare providers and health insurance plans, especially if the content is perceived as culturally insensitive, inaccurate, or simply doesn’t apply to the patients’ specific situations.
For example, if a health plan puts out dietary recommendations based on a standard American diet, and this is simply translated with machine translation, then patients who don’t eat a standard American diet may not understand the recommendations or how to apply them. How can they trust your recommendations if those recommendations have little to do with their daily lives? For example, the diet of a refugee family from Afghanistan is vastly different from a typical American diet.
Lack of trust impacts patient adherence to preventive care recommendations and treatment plans, resulting in poorer outcomes.
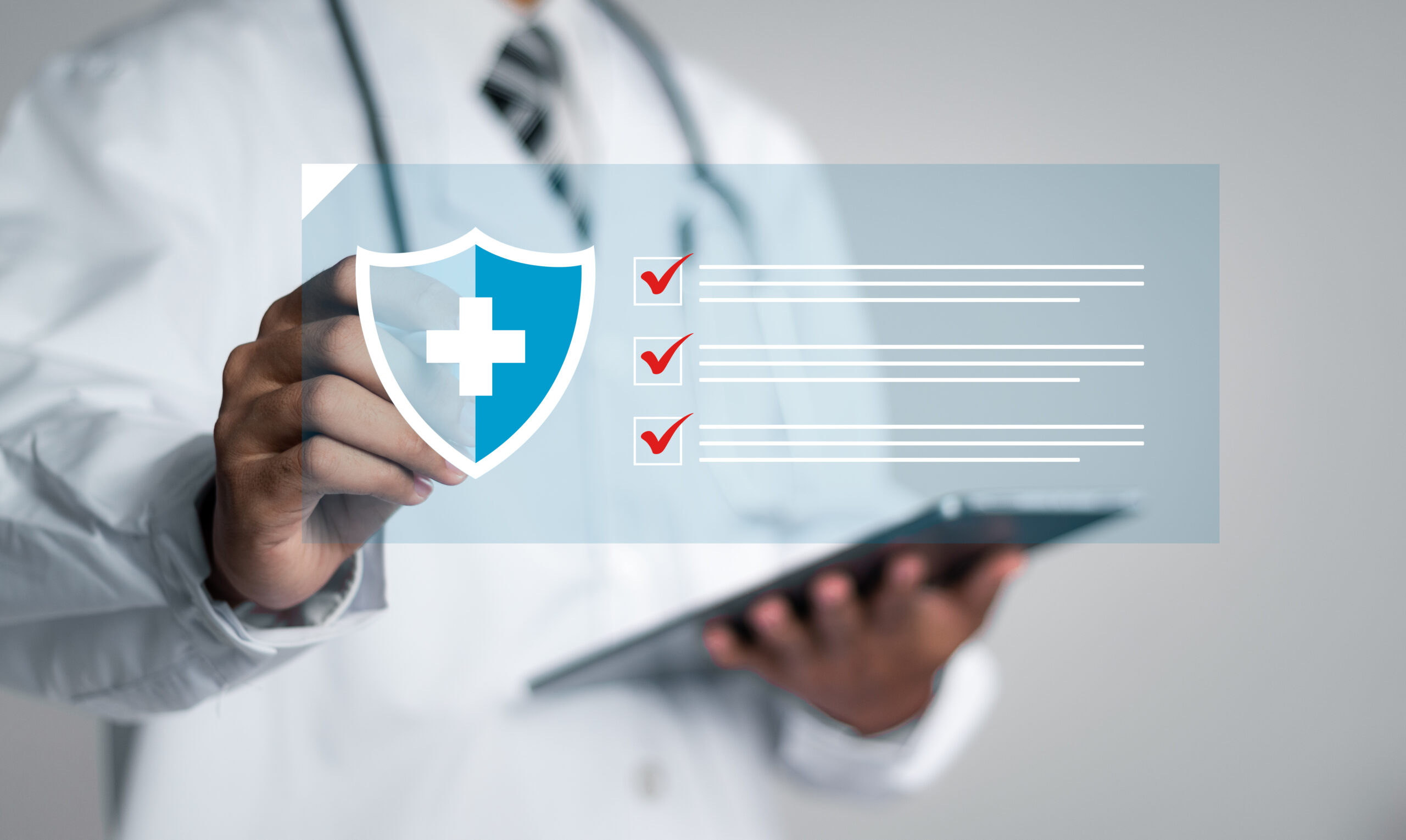
moving towards cultural sensitivity and accuracy in AI translations
AI offers unparalleled speed and efficiency, but the problematic and unresolved fact is that the data that powers it is full of biases and so the outputs are as well. The industry is working hard to resolve these issues, but we aren’t there yet.
In truth, bias in AI translations is the result of a number of overlapping causes such as human bias (in the translator, the post-editor, the prompt engineer, and the AI model engineer), training data bias, algorithmic bias, and the intrinsic bias in languages. Together, these realities drive biased results.
For now, healthcare organizations using AI translation must have a strong post-editing process in place. In this important step, trained linguists review AI-produced output to catch and correct AI-produced errors and biases. These experts understand how cultural bias manifests in AI translations and know how to fix it. However, if all content must be reviewed this process is expensive and time-consuming.
Moving forward, bias in the AI translation process will be mitigated by human expertise, strong quality assurance processes, and customized tools. With this trio of approaches, organizations will achieve the benefit of faster, more scalable translations without the risks of inaccuracy and bias.
Sayegh says, “At CQ fluency, we are committed to eliminating bias from translations and are establishing protocols, processes, and tools to help make sure translations accurately align with the preferences, beliefs, and habits of the target culture.”