Knowing what patients think about the care they receive and the services they need is essential in healthcare. This type of feedback is known as sentiment analysis or opinion mining. It goes beyond patient satisfaction, since it aims to uncover all aspects related with the journey, from before their initial appointment to their final contact with the healthcare provider.
The patient’s journey is often filled with mixed emotions and different impressions, and each touch point is important to improve the patient experience, from the ease of use of an app to schedule an appointment, to interactions with medical professionals and even medication side effects. By gathering and analyzing information about the various pieces that make up the patient experience, companies can focus on the areas that need improvement and keep encouraging practices that result in positive outcomes, thus promoting patient-centered healthcare delivery.
how to analyze patient sentiment
Patient feedback is very subjective, so it is better to collect information through surveys and open-ended questions. Although this allows for deeper insights, it also creates a large amount of unstructured data, with many variables to be accurately measured and managed. Before the advent of modern powerful computing capacity, sentiment analysis was used in written paper documents, which had to be individually read, categorized, and analyzed by a human being. Today, it is possible to leverage artificial intelligence (AI) methods, such as natural language processing (NLP) to perform all tasks involved in sentiment analysis.
There are three important steps in sentiment analysis: identify, extract, and analyze. The goal is to condense complex information and understand if the sentiment behind it is positive, negative, or neutral.
Identifying sources of patient experiences may be somewhat easy, but sentiments are usually scattered in different places, such as proactively collected surveys, reviews placed in online platforms, and opinions shared in social media accounts. This creates a challenge for companies that want to leverage sentiment analysis, because they need to gather considerable amounts of unstructured data from mismatched sources.
After gathering the data, there is a need to organize it and extract the relevant elements for analysis. After being properly categorized, the information can be separated by the sentiment behind it. If we go one step further, it is also possible start to anticipate intentions based on the most common type of sentiment encountered. For example, if most people have negative sentiments towards the task “scheduling appointments,” then this is something that may be preventing them from doing so.
As we have seen, patient sentiment analysis may be time-consuming and challenging to do, since there is a large amount of data to be processed. Fortunately, this can be facilitated using AI.
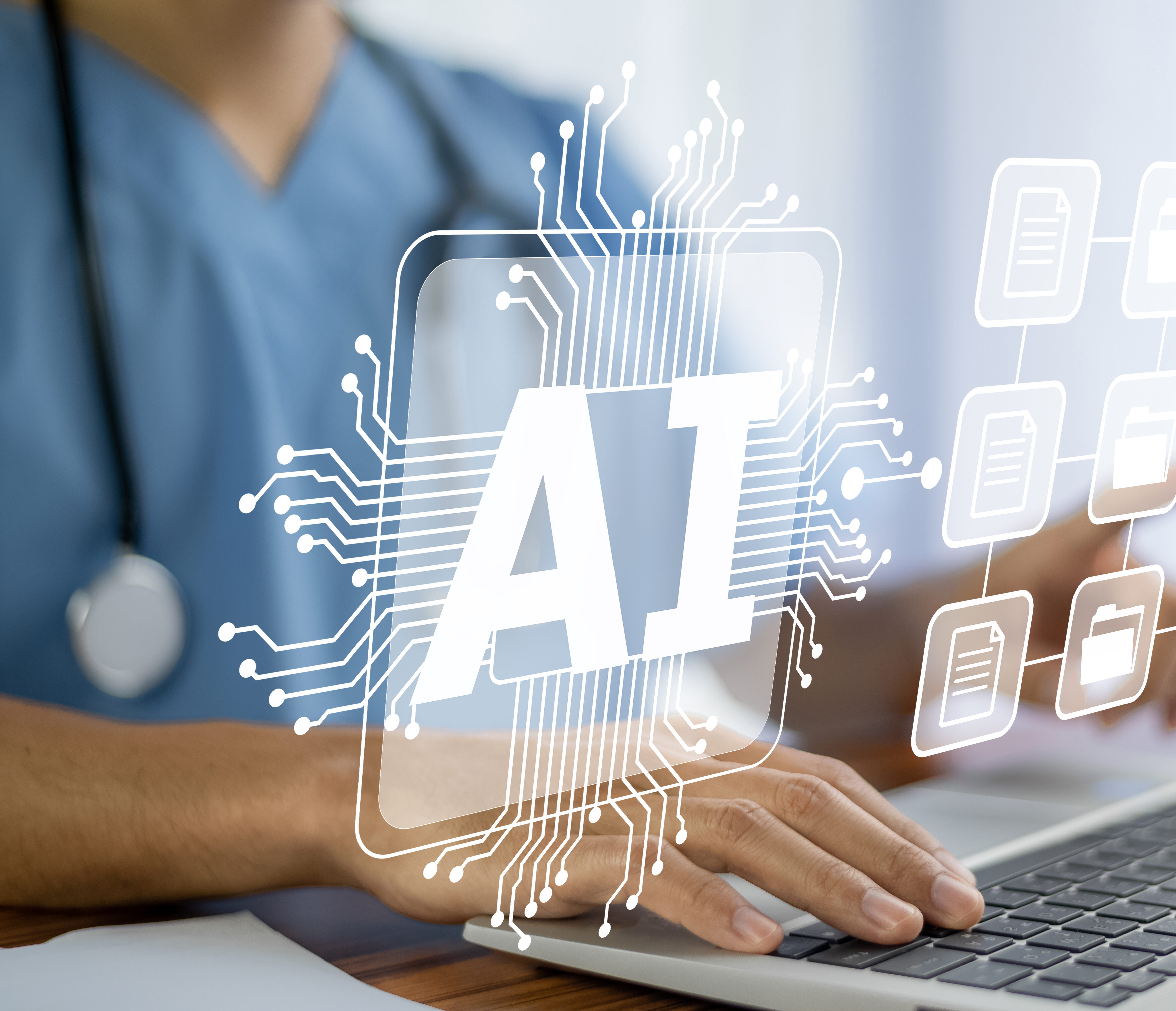
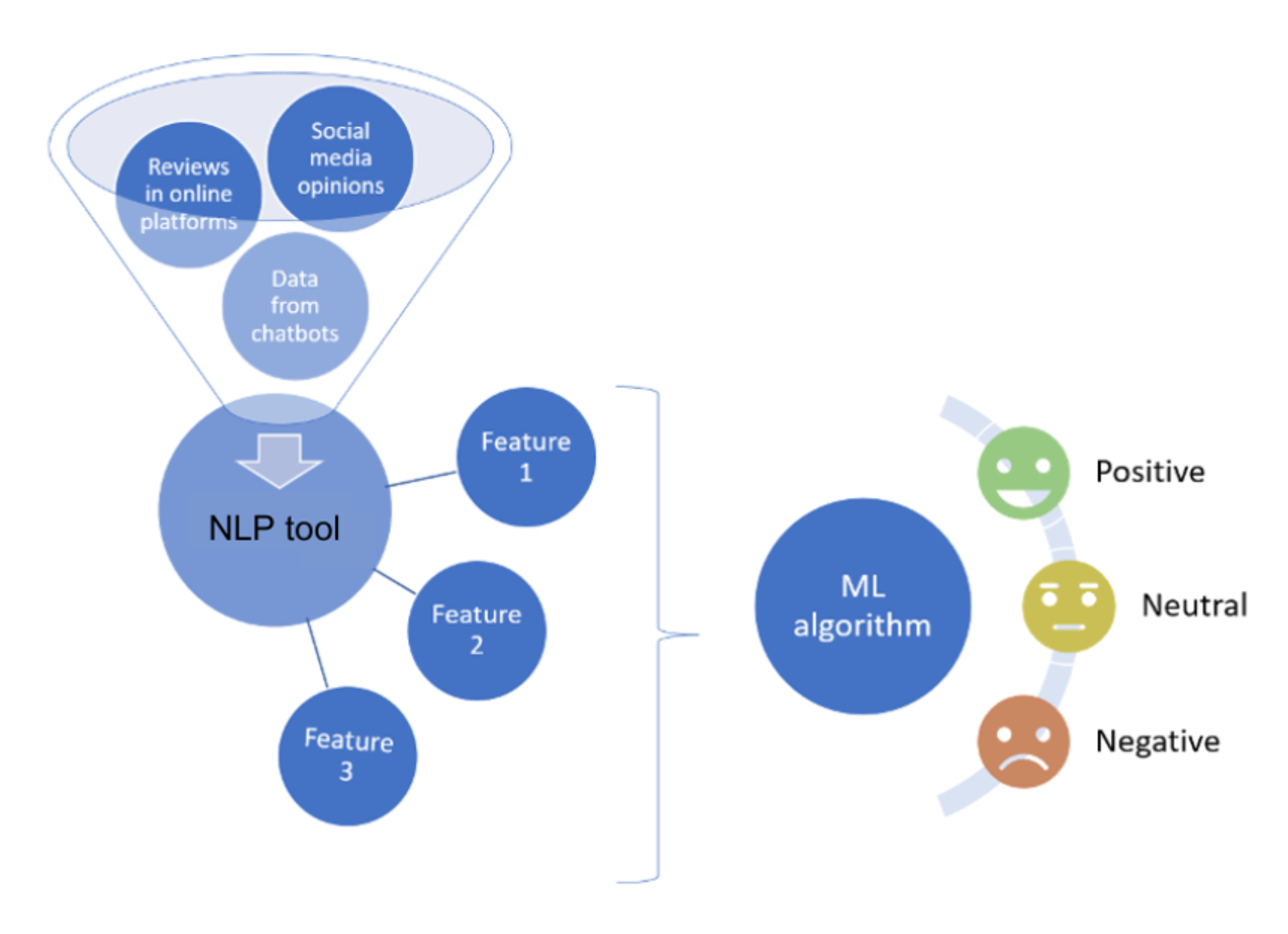
leveraging AI to analyze patient sentiment
Given its ability to process large quantities of data in a structured way, AI tools such as conversational AI (including chatbots), NLP, and machine learning (ML) are ideal to perform sentiment analysis. These tools can be used in isolation, but using them in combination will result in more powerful insights.
Here is an example of how these tools can be used to analyze patient sentiment: after collecting data from several sources with an AI-leveraged aggregator and complementing it with insights from the company’s chatbot, NLP is used to understand the structure of the individual pieces of text and identify its meaning. Then, the features extracted by the NLP tool are fed into an ML algorithm, which classifies them into positive, neutral, or negative.
The insights collected through patient sentiment analysis can be used to improve areas of friction between patients and the healthcare provider, though the analysis of polarization. This is the most known and used form of sentiment analysis. However, more sophisticated AI techniques can also detect emotions in text, taking into account cultural issues, nuances, or sarcasm.
Another form of sentiment analysis can pinpoint aspects of a service or treatment to which patients are placing more importance to. This may be especially relevant for new services or treatment paths, since patients may be giving importance to unforeseen elements. This is important not only for healthcare service providers but also in clinical trials.
AI-driven patient sentiment analysis in clinical trials
Clinical trials are becoming increasingly complex, many with intricate designs and elaborate assessments. This causes not only an increase in cost, but also additional challenges in patient retention. According to an article published in Trends in Pharmacological Sciences, two of the key factors that can cause a clinical trial to fail are ill-suited patient selection and lack of technical infrastructure to monitor patients, thus improving adherence. The authors explain that recruiting the right patients for any given clinical trial is a substantial investment of time and funding. Return on this investment is only possible if the trial is successfully completed, and for this, it is paramount that patients stay in the trial until the end (or for as long as possible) and adhere to trial procedures. However, the average patient dropout rate across clinical trials is 30%, and non-adherence after 150 days is even higher, at 40% on average.
To avoid patient dropout and increase adherence, clinical trial sponsors can leverage AI tools in combination with wearable devices to continuously collect and analyze patient data. The data collected though the wearable device can be complemented with patient surveys. ML algorithms can then analyze the data, identifying patient sentiment patterns and highlighting those that may be at risk of dropping out of a clinical trial, by detecting the onset of behavior that may suggest that the patient is having issues with medication side effects, for example.
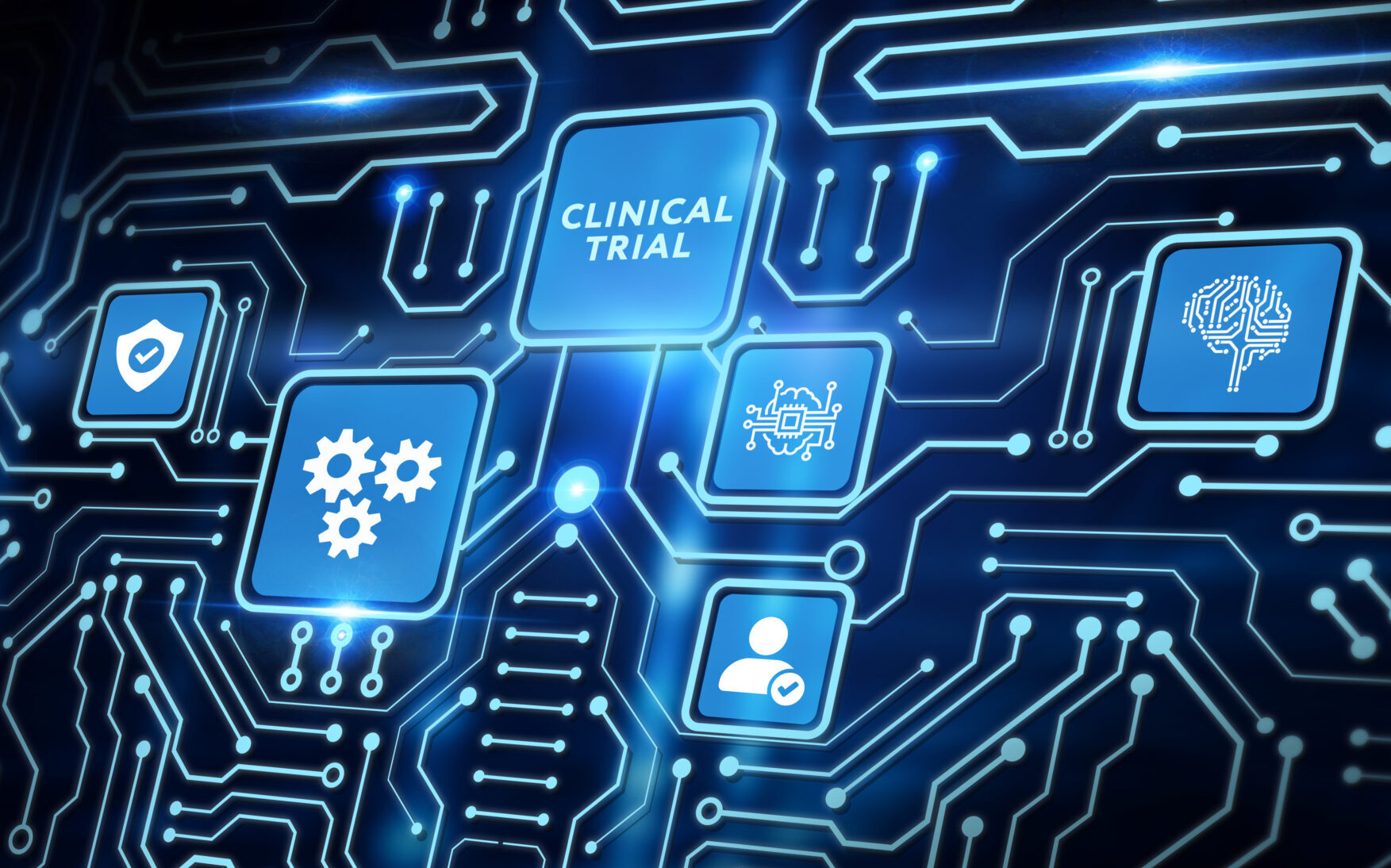
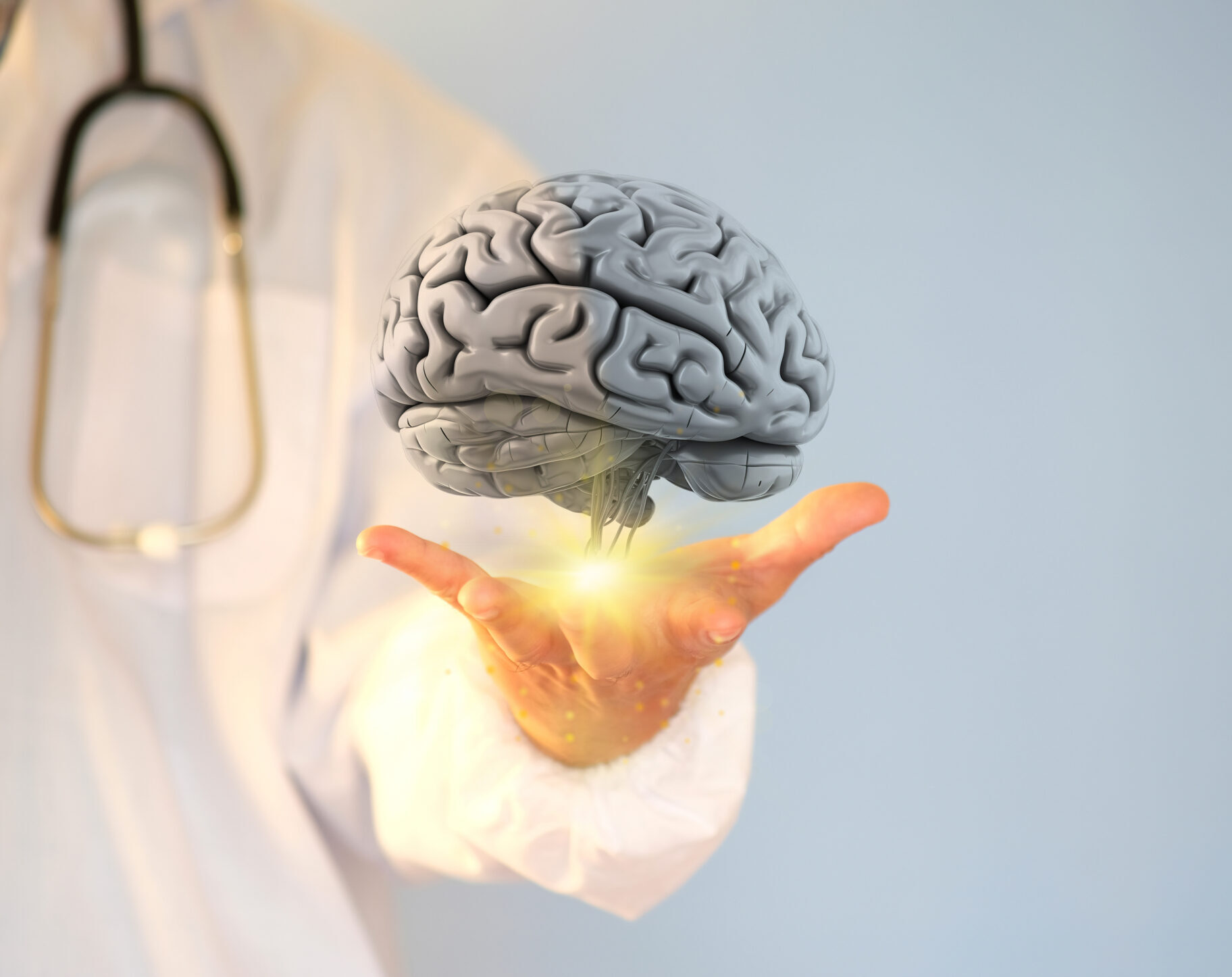
technology experts
In conclusion, AI-enabled patient sentiment analysis is a powerful way to improve service delivery in healthcare and increase clinical trial effectiveness. In the future, stakeholders in healthcare will continue to align their decisions with the patient’s needs. While AI is yet to be adopted by all companies, it has the potential to transform healthcare delivery, and the early adopters of these technologies will be the first to reap their successes. CQ fluency already leverages capable and robust AI solutions, as you can see here. Contact us to see how our advanced technology can assist you.